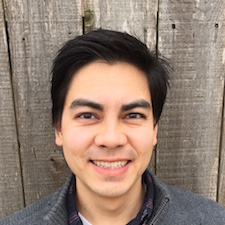
Alexander D'Amour
Staff Research Scientist, Google DeepMind
Cambridge, MA
alexdamour@google.com
I am a Staff Research Scientist at Google DeepMind in Cambridge, MA. Formerly, I was a Neyman Visiting Assistant Professor in the Department of Statistics at UC Berkeley. I did my PhD in the Department of Statistics at Harvard University, where I was advised by Edoardo Airoldi.
I work primarily on problems in causal inference, distribution shift, domain generalization, and fairness for building reliable, trustworthy Machine Learning/AI systems. More generally, I am interested in problems where simple prediction is not enough.
I am interested in all kinds of applications. In research and in consulting, I've worked on problems in sports, healthcare, education, social network analysis, marketing, finance, microfinance, and entertainment.Papers
Note: This section is no longer maintained. Please see Google Scholar for up-to-date publications.
To Appear
On Multi-Cause Causal Inference with Unobserved Confounding: Counterexamples, Impossibility, and Alternatives
Contrary to some recent claims, evaluating many causes (e.g., treatments) simultaneously cannot eliminate unobserved confounding in observational causal inference. This paper demonstrates this point by counterexample
Alexander D'Amour
To appear in Proceedings of AISTATS 2019
Flexible sensitivity analysis for observational studies without observable implications
In causal inference, sensitivity analysis is meant to probe unidentifiable assumptions that are necessary for causal identification, but may sensitivity analysis methods inadvertently impose restrictions that have observable implications. We propose a flexible, interpretable framework that does not have this problem and is compatible with modern observed data modeling techniques.
Alexander Franks, Alexander D'Amour, and Avi Feller
To appear in the Journal of the American Statistical Association
Under Review
Overlap in Observational Studies with High-Dimensional Covariates
In high dimensions, overlap is a stronger assumption than most people realize. This paper presents some implications.
Alexander D'Amour, Peng Ding, Avi Feller, Lihua Lei, and Jasjeet Sekhon
Published
Reducing Reparameterization Gradient Variance
Control variate technique for reducing the variance of stochastic gradients used in Monte Carlo variational inference.
Andrew C. Miller, Nicholas J. Foti, Alexander D'Amour, and Ryan P. Adams
Advances in Neural Information Processing Systems (NIPS), 2017
Meta-Analytics: Tools for Understanding the Statistical Properties of Sports Metrics
Introduces an ensemble of r-squared-style statistics to quantify the reliability and uniqueness of sports metrics.
Alexander Franks, Alexander D'Amour, Daniel Cervone, and Luke Bornn
Journal of Quantitative Analysis in Sports
A Multiresolution Stochastic Process Model for Predicting Basketball Possession Outcomes
Methodology for computing Expected Possession Value, an instantaneous expected point value for a basketball possession.
Daniel Cervone, Alexander D'Amour, Luke Bornn, and Kirk Goldsberry
Journal of the American Statistical Association
Disambiguation and Co-authorship Networks of the U.S. Patent Inventor Database
A supervised learning approach to adding unique inventor identifiers to the US patent database.
G. Li, R. Lai, Alexander D'Amour, D. Doolin, Y. Sun, V. Torvik, A. Yu, and L. Fleming
Research Policy, 2014.
Estimating Rates of Carriage Acquisition and Clearance and Competitive Ability for Pneumococcal Serotypes in Kenya With a Markov Transition Model
Markov model approach to estimating epideiological properties of Pneumococcal serotypes using periodic testing data from Kenyan schoolchildren.
M. Lipsitch, O. Abdullani, Alexander D'Amour, W. Xie, D. Weinberger, E. Tchetgen, and J. Scott
Epidemiology, 2012.
Improving Major League Park Factor Estimates
An ANOVA approach to estimating park factors in Major League Baseball. Written in conjunction with the Harvard Sports Analysis Collective.
R. Acharya, A. Ahmed, Alexander D'Amour, H. Lu, C. Morris, B. Oglevee, A. Peterson, and R. Swift
Dissertation
The Effective Estimand
A framework for characterizing the scientific usefulness of an estimator derived from a misspecified model.
Generalizes the work on networks to general modeling tasks.
Alexander D'Amour and Edoardo Airoldi
- In preparation.
- Working Draft
Misspecification, Sparsity, and Superpopulation Inference for Sparse Social Networks
Theoretical characterization of how the sparse scaling of social networks undermines superpopulation investigations when the sparsity is not modeled exactly.
Proposes sparsity-invariant modeling and inference methodology.
Alexander D'Amour and Edoardo Airoldi
- In preparation.
- Working Draft
- Slides
Causal Inference with Sparse Social-Interaction-Valued Outcomes
Extension of sparsity-invariant methodology for network data to causal settings.
Alexander D'Amour and Edoardo Airoldi
- In preparation.
- Working Draft
Talks, Posters, Other Media
Talks
Overlap in High Dimensions
Surprisingly strong implications of the overlap assumption that is usually invoked in high-dimensional causal inference. Upshot: in high dimensions, the overlap assumption approaches a balance assumption.
Invited talk at the Berkeley Division of Biostatistics Seminar, October 2017 at UC Berkeley.
Invited talk at the Atlantic Causal Inference Conference, May 2017 at UNC Chapel Hill.
Advances in Basketball Analytics Using Player-Tracking Data
High-level overview of new quantiative methods for understanding basketball, implemented by XY Research group using player-tracking data from the NBA.
Invited talk at Consortium for Data Analytics and Risk, October 2017 at UC Berkeley.
Invited talk at Boston ML meetup, July 2016 in Boston, MA.
Prediction is Not Enough: Designing decision-support statistics for causal inference and attribution
Exploration of Statistical applications where the objective requires more than the ability to predict future replications of the observe data stream.
Invited talk at Clarify Health Solutions in San Francisco, CA.
Invited talk at Lumos Labs in San Francisco, CA.
A Design-Based Perspective on Variable Selection
An approach to variable selection that treats it as the design choice -- namely choosing which conditional distribution to model. Some preliminary thoughts on optimal data-splitting.
Talk given in the Harvard Statistics Department's Research in Statistics student colloquium.
Posters
Extrapolation Parameterizations for Assessing Sensitivity to Unmeasured Confounding
Proposes the extrapolation factorization for sensitivity analysis in causal inference, which explicitly separates identified and unidentified parts of the potential outcomes model.
Move or Die: How Ball Movement Creates Open Shots in the NBA
Uses summaries of a Markov model for basketball possessions to show that ball movement is effective only inasmuch as it introduced unpredictability into an NBA offense.
Winner: Best Poster, 2015 Sloan Sports Analytics Conference.
Popular Media
Bayesian Statistician
You're the Expert (radio show)
Behind Databall: A Discussion on the Methodology of Expected Possession Value
Grantland
Teaching
Classes
At Berkeley, I have taught the following courses:
- Statistics 153: Timeseries Analysis (Spring 2017, Fall 2017)
- Statistics 298, 278B: Causal Inference Reading Group (Fall 2016, Spring 2017, Fall 2017, Spring 2018)
- Statistics 88: Probability and Mathematical Statistics for Data Science (Fall 2016)
At Harvard, I was a teaching fellow for the following courses:
- Statistics 220: Bayesian Data Analysis (Fall 2011, Fall 2012)
- Statistics 221: Statistical Computation and Visualization (Spring 2013)
- Statistics 225: Spatial Statistics (Spring 2014)
- Statistics 121/Computer Science 109: Data Science (Fall 2013, Fall 2014)
- Statistics 107: Financial Statistics (Spring 2012)
Awards
- 2014 David Pickard Memorial Teaching Fellow.
- Four-time awardee of the Certificate for Distinction in Teaching.
Consulting
In a previous life, I fielded many applied statistical problems from industry in a Data Science consulting practice. I was a founding partner of Damyata, LLC, a consultancy that I founded with two tech industry veterans. Our mission was to establish best practices in Data Science by delivering state-of-the art data-driven systems to our clients. A core part of our mission was to foster academic-industry research partnerships.
Former consulting clients include